Background
In the context of network offense and defense,The common network security concept is often limited to gateway level, boundary level, such as NGFW, AV, IDPS, etc., important security facilities are generally concentrated in the machine room or at the network entry, and under the strict monitoring of these devices, security threats from the external network are greatly reduced. Conversely,The security threats from the internal network are a common issue reflected by many security managers.
To protect internal network security, some units physically isolate the internal network from the external network, or internally access the external network through a unified gateway, and set up security devices such as firewalls and IDPS at the gateway. Although the aforementioned types of security measures have been implemented, many managers are still troubled byThe frequent occurrence of leak incidents or other types of internal network security incidents fully demonstrates the complexity and importance of internal network security protection in offensive and defensive confrontations.
Mainstream technology
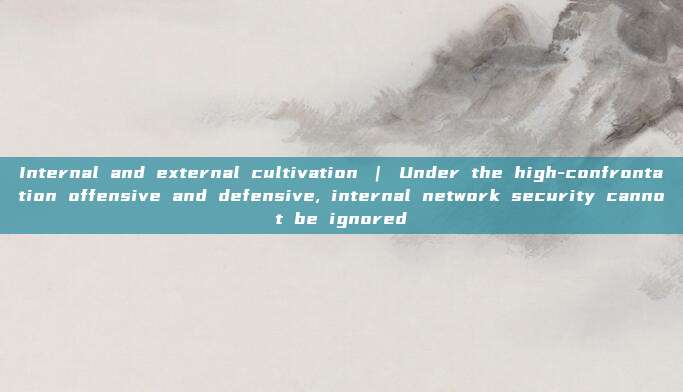
Highly confrontational offensive and defensive measures are conducive to evolving passive defense mechanisms into active defense.Ensure internal network security, it is undoubtedly a key measure in the offensive and defensive confrontation. In the new era of network security, it is necessary to deploy deep defense and offensive and defensive strategies. Through intelligent technologies with behavioral analysis and rapid response, it is essential to discover and eliminate compromised hosts within the internal network and threats in the first time, which is the core essence of internal network security. Internal network protection should be more focused on analyzing the behavior of users and entities, focusing on internal anomalies to discover and eliminate a large number of high-risk risks,The core technology can be positioned in the machine learning dimension based on traffic analysis, data modeling, and model matching, as well as the perspective of high-precision threat determination.
Machine learning and data modeling
On the one hand, toInternal network mutual visit traffic analysis, while establishing data behavior models, analyze individual behavior from multiple dimensions in time series and spatial domains,not only analyze individuals, but also analyze group behavior, establish group baseline and individual baseline based on behavioral characterization and correlation analysis data; with the help of average values, variances, similarity, etc., compare individual and group behaviorIdentifying behaviors that deviate from the normal baseline. Based onThe results of traffic analysis, data modeling, and model matching are used to determine threats on internal network hosts through machine learning,learn normal traffic and optimize the baseline. At the same time, predefined feedback inputs are made to malicious traffic samples. For example, in the laboratory environment, engineers analyze the behavior of millions of malicious software, disassemble it into several tens of malicious behavior categories such as malicious external connections and PE file downloads, and classify them into different behavior families, and then dynamically establish corresponding behavior models. Up to this point,The compromised hosts in the internal network can be captured due to the malicious software infection, and the behavior characteristics that are emitted can be captured.
Precise threat determination
Based onStatic featuresCan efficiently detect qualitative threatsBased on model learningCan quickly make judgments on heterogeneous threats, in addition to the above methods, we foundBy combining intelligence information, the accuracy of threat determination can be greatly improved.
As shown in the figure above, the center representsBased on the known malicious behavior collected and processed through threat intelligence(Predefined negative feedback), the closer the point is to the center, the higher the malicious degree. Various behavior families are divided to determine different security risk levels and harm levels. By matching the traffic and behavior model, precise threat determination can be achieved.
Actual effect
Shan Shi Smart—Intelligent Internal Network Threat Perception System,focusing on the perception of internal network risk situation, committed to the security of core business.
Shan Shi Smart·Focus on key monitoringCore asset server,detect and locate known and unknown network threats, accurately locate risk servers and risk hosts, and fully restore the attack chain behavior, making internal network security detectable, manageable, traceable, and trustworthy.
Real-time monitoring of the dynamic internal network risk situation,penetrate the business applications and interconnection traffic of internal network hosts and servers, visually manage the overall threat impact and mutual visitation relationships of the internal network; discover highly credible known and unknown network attacks and abnormal network behavior, accurately locate compromised hosts, jump hosts, and risk servers.
ThroughNetwork threat tracking, terminal threat tracing, threat knowledge base, risk assessment report, fully grasp the internal network risk situation,providing information support for the identification, disposal, and prediction of internal network risks; filling the gaps in traditional boundary network security construction,联动boundary security devices, forming an overall network security solution of boundary + internal network.
A collection of practical content of security products,联动Cloud Security Product,Boundary Security Product,Security Operation Product,Data Security ProductRead the operation guide together for higher efficiency! Comprehensive and clear product practical explanation is on the way, please look forward to it!
0x01 Entering the Government External Network through the Prefectural Level
Data security from the perspective of the red team in offensive and defensive exercises
A brief discussion on security detection in the context of security attack and defense scenarios
Analysis of the reflective class loading of high-version JDK in practical network defense and attack

评论已关闭