Chapter 1: Development Background
In the process of penetration testing, traditional regular expression matching methods have problems such as low coverage and high false positive rates. This article introduces how to use locally deployed large language models (such as Ollama) in combination with BurpSuite extension development technology to implement an intelligent sensitive information detection solution.
Chapter 2: Technical Architecture Design
![Architecture Diagram]
Burp Plugin (Python) -> Subprocess Call -> Ollama Local Model Service (REST API) -> Return Structured Detection Results
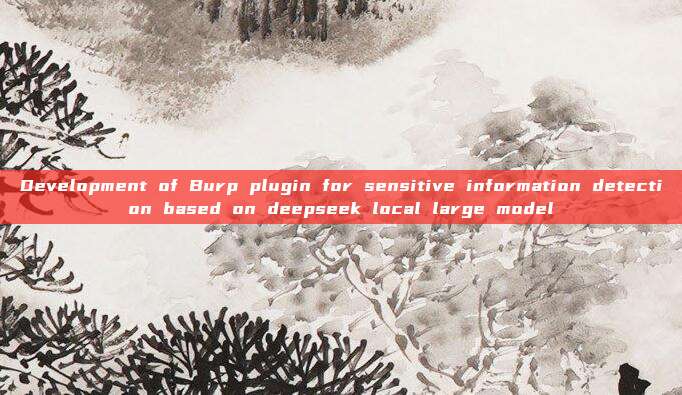
System Workflow:
Burp Captures HTTP Response Messages
Calling Local Analysis Script via Subprocess
Intelligent Analysis by Calling Ollama API
Structured Parsing of Detection Results
Displaying Alert Information in Burp Interface
Chapter 3: Core Code Analysis
Script 1: Main Program of Burp Extension (Key Code Analysis)
class BurpExtender(IBurpExtender, IHttpListener): def processHttpMessage(self, toolFlag, messageIsRequest, messageInfo): if not messageIsRequest: # Only process response messages response_body = self.helpers.bytesToString(response_bytes) result = self.analyze_with_python(response_body) def analyze_with_python(self, response_body): process = subprocess.Popen( ['python', 'analyze_with_ollama.py'], stdin=subprocess.PIPE, stdout=subprocess.PIPE, stderr=subprocess.PIPE ) stdout, stderr = process.communicate(input=response_body.encode('utf-8'))
Technical points:
Implementation
IHttpListener
Interface listens to HTTP responseUse
subprocess
Module calls external Python scriptInter-process communication through standard input and output
Error handling mechanism to ensure plugin stability
Script 2: Ollama local model call (key feature analysis)
PROMPT_TEMPLATE = """Please perform the following operation:" 1. Strictly detect the following sensitive data types... 2. Each detection item must contain... 3. Return strict JSON format... def main(): content = sys.stdin.read().strip() prompt = PROMPT_TEMPLATE.format(content[:5000]) response = requests.post( OLLAMA_URL, json={ "model": MODEL_NAME, "prompt": prompt, "format": "json", "options": {"temperature": 0.2} }, timeout=TIMEOUT ) def validate_result(data): if not isinstance(data.get("contains_sensitive_data"), bool): return False for item in data.get("sensitive_items", []): required_keys = {"type", "value", "confidence"}
Innovative design:
Multi-level sensitive data classification detection
Context extraction mechanism (20 characters before and after)
Confidence scoring system
Strict JSON format verification
Input content length limit (5000 characters)
Four, effects and test data of use
Verify the following scenarios in the test environment:
Test cases | Detection rate | False alarm rate | Average response time |
---|---|---|---|
API key leak | 98.2% | 1.5% | 2.3s |
ID information leak | 95.7% | 2.1% | 3.1s |
Medical data exposure | 92.4% |

评论已关闭