Introduction:
1、The Advanced Email Attacks of Hacker Group Dark Basin
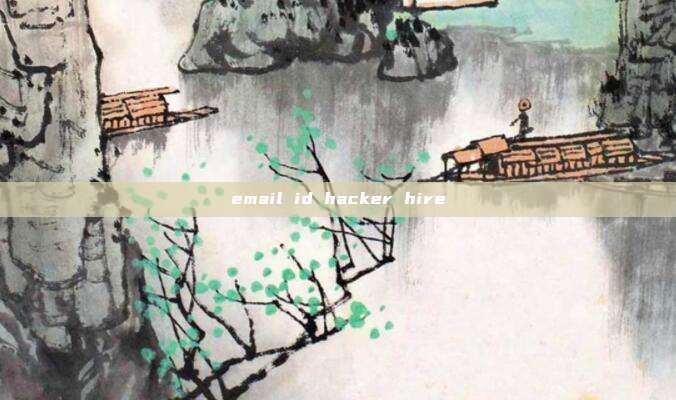
2、‘Dark Basin’ hacking group targeted thousands in hack-for-hire scheme
The Advanced Email Attacks of Hacker Group Dark Basin ♂
For Security Operation Centers (SOCs), reliance on signature or rule-based tools – solutions that are always chasing the latest update to prevent only what is already known – creates an excess of false positives. SOC analysts are therefore overwhelmed by a high volume of context-lacking alerts, with human analysts able to address only about 10% due to time and resource constraints. This forces many teams to accept the risks of addressing only a fraction of the alerts while novel threats go completely missed.
74% of practitioners are already grappling with the impact of an AI-powered threat landscape, which amplifies challenges like tool sprawl, alert fatigue, and burnout. Thus, achieving a resilient network, where SOC teams can spend most of their time getting proactive and stopping threats before they occur, feels like an unrealistic goal as attacks are growing more frequent.
Despite advancements in security technology (advanced detection systems with AI, XDR tools, SIEM aggregators, etc...), practitioners are still facing the same issues of inefficiency in their SOC, stopping them from becoming proactive. How can they select security solutions that help them achieve a proactive state without dedicating more human hours and resources to managing and triaging alerts, tuning rules, investigating false positives, and creating reports?
To overcome these obstacles, organizations must leverage security technology that is able to augment and support their teams. This can happen in the following ways:Full visibility across the modern network expanding into hybrid environmentsHave tools that identifies and stops novel threats autonomously, without causing downtimeApply AI-led analysis to reduce time spent on manual triage and investigation
Traditional cybersecurity point solutions are reliant on using global threat intelligence to pattern match, determine signatures, and consequently are chasing the latest update to prevent only what is known. This means that unknown threats will evade detection until a patient zero is identified. This legacy approach to threat detection means that at least one organization needs to be ‘patient zero’, or the first victim of a novel attack before it is formally identified.
Even the point solutions that claim to use AI to enhance threat detection rely on a combination of supervised machine learning, deep learning, and transformers to
train and inform their systems. This entails shipping your company’s data out to a large data lake housed somewhere in the cloud where it gets blended with attack data from thousands of other organizations. The resulting homogenized dataset gets used to train AI systems — yours and everyone else’s — to recognize patterns of attack based on previously encountered threats.
While using AI in this way reduces the workload of security teams who would traditionally input this data by hand, it emanates the same risk – namely, that AI systems trained on known threats cannot deal with the threats of tomorrow. Ultimately, it is the unknown threats that bring down an organization.
Enter Extended Detection and Response (XDR): a platform approach aimed at unifying threat detection across the digital environment. XDR was developed to address the limitations of traditional, fragmented tools by stitching together data across domains, providing SOC teams with a more cohesive, enterprise-wide view of threats. This unified approach allows for improved detection of suspicious activities that might otherwise be missed in siloed systems.
However, XDR solutions still face key challenges: they often depend heavily on human validation, which can aggravate the already alarmingly high alert fatigue security analysts experience, and they remain largely reactive, focusing on detecting and responding to threats rather than helping prevent them. Additionally, XDR frequently lacks full domain coverage, relying on EDR as a foundation and are insufficient in providing native NDR capabilities and visibility, leaving critical gaps that attackers can exploit. This is reflected in the current security market, with 57% of organizations reporting that they plan to integrate network security products into their current XDR toolset[1].
The result of these shortcomings within the security solutions market is an acceptance of inevitable risk. From false positives driving the barrage of alerts, to the siloed tooling that requires manual integration, and the lack of multi-domain visibility requiring human intervention for business context, security teams have accepted that not all alerts can be triaged or investigated.
While prioritization and processes have improved, the SOC is operating under a model that is overrun with alerts that lack context, meaning that not all of them can be investigated because there is simply too much for humans to parse through. Thus, teams accept the risk of leaving many alerts uninvestigated, rather than finding a solution to eliminate that risk altogether.
Darktrace / NETWORK is designed for your Security Operations Center to eliminate alert triage with AI-led investigations , and rapidly detect and respond to known and unknown threats. This includes the ability to scale into other environments in your infrastructure including cloud, OT, and more.
Darktrace does not rely on known malware signatures, external threat intelligence, historical attack data, nor does it rely on threat trained machine learning to identify threats.
Darktrace’s unique Self-learning AI deeply understands your business environment by analyzing trillions of real-time events that understands your normal ‘pattern of life’, unique to your business. By connecting isolated incidents across your business, including third party alerts and telemetry, Darktrace / NETWORK uses anomaly chains to identify deviations from normal activity.
The benefit to this is that when we are not predefining what we are looking for, we can spot new threats, allowing end users to identify both known threats and subtle, never-before-seen indicators of malicious activity that traditional solutions may miss if they are only looking at historical attack data.
Anomaly detection is often criticized for yielding high false positives, as it flags deviations from expected patterns that may not necessarily indicate a real threat or issues. However, Darktrace applies an investigation engine to automate alert triage and address alert fatigue.
Darktrace’s Cyber AI Analyst revolutionizes security operations by conducting continuous, full investigations across Darktrace and third-party alerts, transforming the alert triage process. Instead of addressing only a fraction of the thousands of daily alerts, Cyber AI Analyst automatically investigates every relevant alert, freeing up your team to focus on high-priority incidents and close security gaps.
Powered by advanced machine-learning techniques, including unsupervised learning, models trained by expert analysts, and tailored security language models, Cyber AI Analyst emulates human investigation skills, testing hypotheses, analyzing data, and drawing conclusions. According to Darktrace Internal Research, Cyber AI Analyst typically provides a SOC with up to ?50,000 additional hours of Level 2 analysis and written reporting annually, enriching security operations by producing high level incident alerts with full details so that human analysts can focus on Level 3 tasks.
Simply quarantining a device is rarely the best course of action - organizations need to be able to maintain normal operations in the face of threats and choose the right course of action. Different organizations also require tailored response functions because they have different standards and protocols across a variety of unique devices. Ultimately, a ‘one size fits all’ approach to automated response actions puts organizations at risk of disrupting business operations.
Darktrace’s Autonomous Response tailors its actions to contain abnormal behavior across users and digital assets by understanding what is normal and stopping only what is not. Unlike blanket quarantines, it delivers a bespoke approach, blocking malicious activities that deviate from regular patterns while ensuring legitimate business operations remain uninterrupted.
Darktrace offers fully customizable response actions, seamlessly integrating with your workflows through hundreds of native integrations and an open API. It eliminates the need for costly development, natively disarming threats in seconds while extending capabilities with third-party tools like firewalls, EDR, SOAR, and ITSM solutions.
Securing the network isn’t just about responding to incidents — it’s about being proactive, adaptive, and prepared for the unexpected. The NIST Cybersecurity Framework (CSF 2.0) emphasizes this by highlighting the need for focused risk management, continuous incident response (IR) refinement, and seamless integration of these processes with your detection and response capabilities.
Despite advancements in security technology, achieving a proactive posture is still a challenge to overcome because SOC teams face inefficiencies from reliance on pattern-matching tools, which generate excessive false positives and leave many alerts unaddressed, while novel threats go undetected. If SOC teams are spending all their time investigating alerts then there is no time spent getting ahead of attacks.
Achieving proactive network resilience — a state where organizations can confidently address challenges at every stage of their security posture — requires strategically aligned solutions that work seamlessly together across the attack lifecycle.
?References
‘Dark Basin’ hacking group targeted thousands in hack-for-hire scheme ♂
Dubbed Dark Basin in?a report?into the group released today by The Citizen Lab, the group is tied to Indian company BellTrox InfoTech Services Pvt Ltd. It’s believed to have targeted advocacy groups and journalists, elected and senior government officials, hedge funds and multiple industries.
Although?the clients of the hacking group are unknown, targets are said to include equity giant KKR, short seller Muddy Waters Research and #ExxonKnew, a campaign against ExxonMobil. Dark Basin is also linked to phishing campaigns targeting organizations that work on net neutrality advocacy.
Phishing is key to the hacking campaign with Reuters?reporting?that it had reviewed a cache of data from the hacking group that detailed tens of thousands of malicious emails sent by BellTroX between 2013 and 2020.
BellTrox owner?Sumit Gupta was previously charged in a 2015 hacking case in which U.S. private investigators said that they had paid him to hack the accounts of marketing executives. Gupta denies being involved in hacking, saying that he only assisted private investigators to download messages from email inboxes after being provided with login details.
“The Citizen Lab’s report reads like a movie script,”?Colin Bastable, chief executive officer of security awareness training firm?Lucy Security AG, told SiliconANGLE. “Half the time I’m thinking that the bad guys left so many trails that it must be an exercise in misdirection. Only state actors could pull something like this together.”
He added that “the quality of the phishing site landing pages is excellent and the English grammar is very good — too good, unless you were running a very professional well-financed and targeted operation. The subdomains are also well-designed, especially for mobile users. The URL shorteners, the five-and-a-half-hour time zone difference and the different email address which tie back to BellTroX are all very interesting.”
Chris Rothe, co-founder and chief product officer at threat detection company?Red Canary Inc., noted that although the investigation doesn’t conclusively show any major damage to the targets, it does show the usage of techniques consistent with those used by many hackers attacking corporations and individuals alike. “While there is nothing groundbreaking about the techniques employed, it is a good reminder that attacks can come from all angles including business or political adversaries,” he said.
Paul Bischoff, privacy advocate with tech research firm?Comparitech Ltd., said the most striking part of the Dark Basin operation is how it was able to advertise its services openly without consequence.
Related questions
To solve this problem, we need to determine the number of ways to split an array into three contiguous parts such that each part has the same sum.
Approach
- Check Divisibility: First, check if the total sum of the array is divisible by 3. If not, it's impossible to split the array into three parts with equal sums, so the answer is 0.
- Compute Prefix Sums: Calculate the prefix sums of the array to efficiently determine sums of subarrays.
- Identify Split Points: Identify indices where the prefix sum equals one-third (part_sum) and two-thirds (2 * part_sum) of the total sum. These indices are potential split points for the array.
- Count Valid Splits: For each valid starting split point (where the sum is part_sum), count how many valid ending split points (where the sum is 2 * part_sum) exist after it. This is done using binary search for efficiency.
Solution Code
import bisect
def count_ways_to_split(arr):
n = len(arr)
total = sum(arr)
if total % 3 != 0:
return 0
part_sum = total // 3
prefix = []
current = 0
for num in arr:
current += num
prefix.append(current)
A = []
B = []
for i in range(n):
if prefix[i] == part_sum and i <= n - 3:
A.append(i)
if prefix[i] == 2 * part_sum and i <= n - 2:
B.append(i)
B.sort()
count = 0
for a in A:
pos = bisect.bisect_right(B, a)
count += len(B) - pos
return count
# Example usage:
# arr = list(map(int, input().split()))
# print(count_ways_to_split(arr))
Explanation
- Divisibility Check: If the total sum of the array is not divisible by 3, return 0 immediately.
- Prefix Sums: Compute the prefix sums to quickly access the sum of any subarray starting from the first element.
- Collect Split Points:
A
collects indices where the prefix sum is one-third of the total sum and ensures there are at least two elements left after the split.B
collects indices where the prefix sum is two-thirds of the total sum and ensures there is at least one element left after the split.
- Count Valid Splits: For each valid starting index in
A
, use binary search to count how many valid ending indices inB
come after it. This ensures the splits are contiguous and non-overlapping.
This approach efficiently narrows down potential split points and uses binary search to quickly count valid combinations, resulting in an optimal solution.
Google Launches 'Email Masking' Feature to Help Users Hide Their Email Address
email id hacker hire(Email Grappler)
Enterprise internal phishing email drill guide
From the perspective of the attacker, to build the email security system of the principal party
How to make ConcurrentMapCacheManager support automatic deletion of expired items
Experience sharing on the opening of two-factor authentication for university email systems

评论已关闭