Credit cards are the most common financial credit products, originally intended to meet the needs of users for advance consumption and convenient payment. Issuing institutions grant credit limits to applicants based on their credit qualifications, allowing them to use credit card limits for convenient credit payments in various consumption scenarios. The used credit limits can be restored and reused for more consumption payments after repayment.
Cashing out and card farming are common irregular operations with credit cards
Since not all consumption scenarios supported card payment when credit cards were first introduced, and many places only accepted cash payments, to expand the use scenarios and improve user experience, many credit card products have also opened cash withdrawal services based on credit payment. Usually, the cash withdrawal limit of credit cards does not exceed 30% of the credit limit, and the part overdrawn for cash withdrawal does not have an interest-free period, and the corresponding handling fee and interest are higher than those for card swipe consumption, with higher borrowing costs and is only suitable for short-term emergency situations.
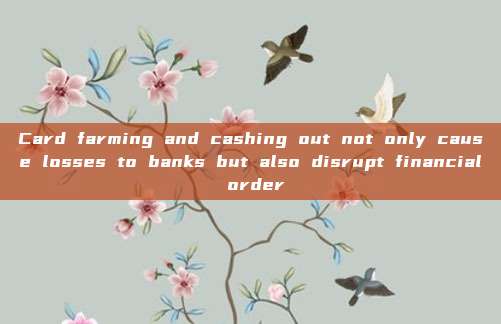
Compared to the credit card cash withdrawal, which has fewer rules and less limit, credit card cashing out is most attractive to fraudulent users. By using illegal merchants or card swipe equipment to create false card swipe consumption transactions, they can convert the entire credit limit into cash with a small handling fee. The methods of cashing out include 'others consuming with their own card', collaborating with merchants or certain 'loan companies', 'intermediary companies' for cashing out, or using some websites or company services for cashing out.
In addition to credit card cashing out, there is also the fraudulent practice of 'card farming with card'. By using a portion of the credit limit of some credit cards through consumption or cashing out, then spending (withdrawing) the remaining balance after the billing date, the money received from consumption can be used to repay the debt, and by repeating this operation, it is possible to achieve the repayment of the billing amount. Moreover, the amount of multiple consumption will appear on the next billing date, and with the help of cashing out, it can achieve an indefinite loan, with only a part of the handling fee to be paid each month. Of course, fraudulent users can also apply for multiple credit cards from different banks to achieve the above 'card farming with card'.
Each credit card transaction directly reflects specific information such as the transaction location and content, which is convenient for the issuing bank to promptly understand the use of credit card funds and potential risks, and is conducive to the macro decision-making of regulators. However, fraudulent behaviors such as card farming and cashing out cannot grasp the flow of funds or understand the user's borrowing purposes, which means the issuing bank has to bear relatively higher credit risks, and these actions are also in violation of regulatory requirements. For example, since last year, the China Banking and Insurance Regulatory Commission has issued multiple notices to strictly control the use of credit card funds for investment, real estate transactions, and other purposes.
The People's Bank of China's 'Overall Operation of Payment System in the Second Quarter of 2019' shows that by the end of the second quarter, the total number of credit cards and integrated cards in use issued was 7.11 billion, with a year-on-year increase of 3.04%. The total credit amount of credit cards was 163.2 trillion yuan, with a year-on-year increase of 3.23%; the balance of credit that should be repaid was 72.3 trillion yuan, with a year-on-year increase of 3.64%. The average credit limit per card was 22.9 thousand yuan, and the credit utilization rate was 44.31%. The total amount of credit that has been overdue for half a year and not repaid was 83.884 billion yuan, accounting for 1.17% of the total credit that should be repaid, with a comparison ratio that rose by 0.02 percentage points from the end of the previous quarter.
Card farming and cashing out not only cause losses to banks but also disrupt financial order
Card farming and cashing out are acts of financial fraud, which not only go against the regulatory agencies' vision of preventing the rapid growth of residents' leverage ratios, but also bring credit risks and financial losses to cardholders. The main hazards are concentrated in the following three aspects:
Firstly, credit card cash withdrawal increases the unstable factors in the financial order. China has strict entry systems for financial institutions and a series of strict regulations to monitor the inflow and outflow of financial institution funds. Unlawful elements, in conjunction with merchants, engage in fictitious POS machine card consumption and other false transactions, which are in violation of the legal framework of the state's regulations on the exclusive operation of financial businesses, deviating from the relevant provisions of the People's Bank of China on cash management, and may provide便利 conditions for illegal activities such as money laundering. This undoubtedly lays unstable factors for the overall financial order in our country. In addition, the formation of a large number of non-performing loans will also damage the social credit environment and hinder the healthy development of the credit card industry.
Secondly, illegal cash withdrawal brings financial losses to issuing banks. The vast majority of credit cards are unsecured lending tools. As long as cardholders make purchases, banks must bear a portion of the repayment risk. Under normal circumstances, banks prevent overdraft risks through high overdraft interest rates or cash withdrawal fees. However, the act of credit card cash withdrawal just avoids the high cash withdrawal fees set by banks and crosses the bank's preventive threshold. Especially some loan intermediaries help cardholders forge identity documents, continuously increase credit card limits, causing great interference to the normal business of banks and bringing huge risks. Due to the large amount of cash withdrawal funds, cardholders obtain personal loans without interest and guarantees. However, issuing banks cannot obtain information about the use of these funds, making it difficult to effectively identify and track them. The credit risk of credit cards has actually evolved into credit risk of investment or speculation. Once cardholders fail to repay the cash withdrawal amount, the losses to banks are not only the interest on the loan but also a large amount of assets.
Thirdly, the act of credit card cash withdrawal brings great credit risk to cardholders. On the surface, cardholders obtain cash through cash withdrawal, reducing interest expenditure, but in essence, cardholders ultimately need to repay. If cardholders fail to repay on time, they must bear interest on overdue repayments that is higher than the interest on overdrafts, and may cause adverse credit records. It will be very difficult to borrow funds from banks in the future, and even bear legal risks of credit loss.
Traditional means of preventing and controlling card-hoarding and cash withdrawal and new challenges
For the identification of card-hoarding and cash withdrawal, traditional prevention and control measures mainly include rule-based and model-based methods.
Based on rule methods: For the transaction behavior and user information of an individual user, rules are generated based on empirical thresholds for the right variables, and then connected into strategies through logical operators.
Based on model methods: A batch of labeled black and white accounts are selected as samples. Personal information and transaction behaviors of these accounts are extracted and derived into features, and then a classification model is trained based on the labels and features of the samples, such as LR, GBDT, and neural network models, etc. Finally, the classification model trained on historical data is applied to new unlabeled accounts to judge the probability of new accounts being used for card-hoarding and cash withdrawal.
Traditional anti-carding and cashing methods are mainly based on individual user prevention, while credit card fraud today is more complex and variable, with characteristics such as long time span, gang-like nature, and concealment, which brings many new challenges to financial institutions in anti-fraud efforts.
1. Carding and cashing often has a gang-like nature, such as illegal merchants providing carding and cashing services using the same phone number to apply for multiple credit cards, or owning multiple POS machines for false transactions. Traditional anti-carding and cashing means are limited to confirming whether a certain account is carding and cashing, and only when a credit card is overdue, it may trace the POS machines involved in the transaction.
2. Anti-carding and cashing methods for single users often require a certain time span and a certain number of transaction records for a single user. If the time span is too short or the number of transactions is too few, it is impossible to hit the rules or derive characteristics. Traditional anti-carding and cashing means are difficult to discover risks at the initial stage of fraud, and by the time risks can be confirmed, multiple transactions have already been made, resulting in losses.
3. Fraudulent users engaging in carding and cashing will use various means to hide their fraudulent behavior and share their experience with others (such as, adjusting the strategy of false transactions, and conducting small-scale cashing below a certain amount threshold), thereby attracting more people to join the carding and cashing camp. Traditional anti-carding and cashing means of identification are based on risks that have already occurred, with strategy rules accumulating relatively lagging, and the speed of risk control is slow, so they cannot prevent and control fraud in a timely and effective manner.
How to effectively prevent and control credit card fraud using the associated network
To prevent and control credit card cashing, it is necessary to start from multiple aspects. In addition to strengthening the management of credit card issuance, review, and post-maintenance, standardizing the credit card marketing assessment mechanism, and strengthening supervision over designated merchants and third-party payment service providers, it is more important to strengthen the management of customer transactions.
1. Based on the transaction relationship between POS machines and credit cards, the binding relationship between credit cards and phone numbers, and the one-degree, two-degree, even three-degree relationships of credit cards in the credit card transaction network, a three-dimensional associated network is established, providing financial institutions with timely and rapid scientific decision-making basis.
2. Quickly identify potential and unknown risks. Once a node (such as: phone number, credit card, POS machine, etc.) is found to have risks, or it is determined that it is carding and cashing, then all nodes related to it may have transmission risks. The associated network quickly locates suspicious transactions and fraudulent activities, helping financial institutions control risks at an early stage.
3. Significantly increase the cost of carding and cashing, making it difficult for fraudulent users to give up. If fraudulent users want to completely hide their fraudulent behavior, they need to use different phone numbers when applying for credit cards, and they must also use different POS machines when cashing out with different credit cards. This may make the cost of carding exceed the benefits of carding and cashing, resulting in a complete loss.
The main steps for preventing and controlling credit card carding and cashing through the associated network are as follows:
Firstly, based on application information and POS machine and other transaction device information, an associated network for credit cards is established. Among them, the payment node can be one of the types such as credit card account number, credit card number, and credit card, and the receiving node can be POS machine ID and merchant number, etc.
Second, filter nodes with high node degrees and filter out some transactions that are too small.
Third, calculate the risk value of nodes and label known cashback nodes.
Fourth, through iterative algorithms, effectively propagate the risk value in the network and explore more potential credit card accounts.
Fifth, feedback the results to the business department to confirm the credit card accounts with the highest risk value.
With the help of the associated network, a certain bank uncovered thousands of credit cards with risky transactions. After investigating the top 1000 high-risk credit cards one by one, it was found that about 80% of them were confirmed to be cashback users.
Neglected Self-owned Data
Last month, regulators conducted a整顿 of companies involved in illegal web scraping of user data. This has affected multiple financial institutions that heavily rely on third-party data for pre-loan approval, risk control approval, and credit limit calculation, leading them to urgently adjust their risk control processes, with some even halting credit card and online credit business.
In addition, more financial institutions and fintech companies are reviewing their senior business and products to determine if they involve illegal web scraping or support from unknown third-party data sources, and they are beginning to think about how to enhance the resilience of their risk control systems to cope with market changes and sustain business development.
In fact, most financial institutions with online businesses accumulate a large amount of user application data, product operation data, and transaction behavior data after conducting related business for a certain period of time. These data are scattered in various disjointed systems in most financial institutions, in addition to being summarized in some coarse-grained business status statistics reports, and most of them are ignored. Not only is the huge value not fully exploited and utilized, but it is also a great waste.
A white paper released by IDC this spring revealed that approximately 7.6ZB of data was generated domestically in 2018, and it is expected that this number will increase to 48.6ZB by 2025. However, only 2.5% of the data has been analyzed and utilized, with 97.5% of the data still dormant.
The associated network can fully utilize the data owned by financial institutions, using graph computing, traditional machine learning algorithms, and even cutting-edge deep learning models to explore risk points within the associated network. It fully taps into the 'data gold mine' of massive data such as internal customer characteristics, business data, transaction information, core credit information, and compliance data, based on a deep understanding of the specific business scenarios, business logic, product processes, customer characteristics, and risk characteristics of financial institutions. It scientifically constructs a complex associated network with 'connotation' and 'extensibility'. Furthermore, through multi-angle exploration using graph data mining, unsupervised algorithms, semi-supervised algorithms, and supervised algorithms, it combines application scenarios and the specific needs of operational personnel to present the most effective information intuitively and intelligently on the operation and monitoring platform, thereby providing anti-fraud, precise marketing, and refined operation services for multiple industries and scenarios, helping to innovate and grow the business.
*Original Author: blackhole666, This article is part of the FreeBuf Original Reward Program, reproduction is prohibited without permission

评论已关闭